In the rapidly evolving landscape of artificial intelligence, the demand for Custom Fine-Tuned Large Language Models (LLMs) is surging. These advanced models are designed to meet specific business requirements, offering unparalleled language understanding and enabling sophisticated automation capabilities. By leveraging state-of-the-art natural language processing (NLP) techniques, businesses can create tailored solutions that enhance user experiences, streamline operations, and drive efficiency across various functions.
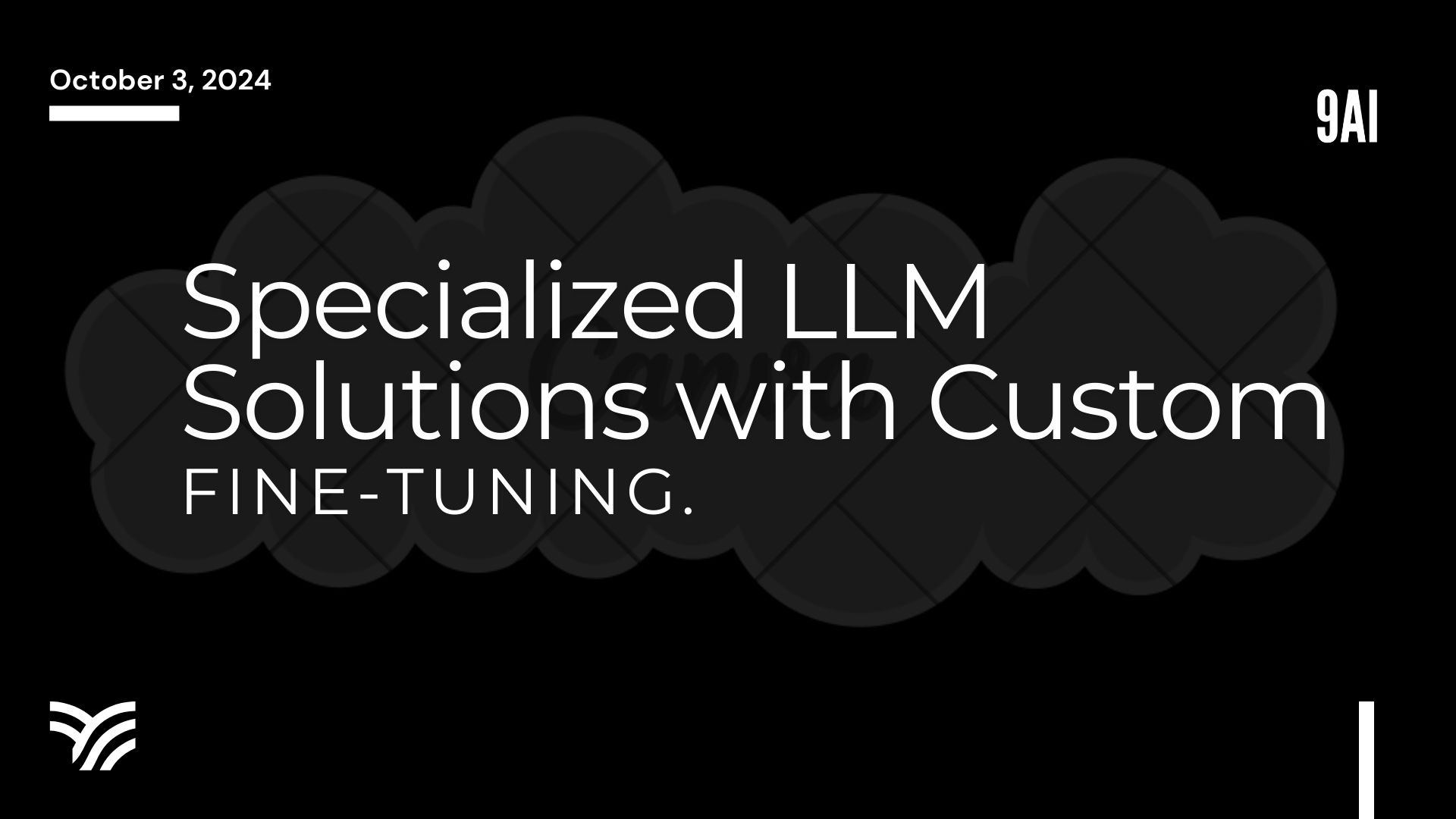
Understanding Large Language Models
Large language models are powerful AI systems trained on vast datasets to understand and generate human-like text. They excel in a variety of tasks, such as language translation, content creation, and sentiment analysis. However, the true potential of these models is realized when they are fine-tuned for specific business use cases, allowing organizations to harness their capabilities more effectively.
1. The Importance of Customization
The off-the-shelf LLMs, while robust, may not fully align with the unique needs of every organization. Custom fine-tuning involves adapting a pre-trained model to better understand the nuances of a specific industry or domain, leading to enhanced accuracy and relevance in responses.
Benefits of Fine-Tuning LLMs
1. Superior Language Understanding
Fine-tuned LLMs are capable of comprehending context-specific language, terminology, and jargon that may be prevalent in a particular business sector. This results in more accurate and contextually appropriate outputs, ensuring that the AI-generated content resonates with target audiences.
2. Enhanced Automation Capabilities
By fine-tuning LLMs, organizations can automate various processes such as customer service, content generation, and data analysis. This not only improves operational efficiency but also reduces the workload on human teams, allowing them to focus on higher-value tasks.
3. Improved User Experience
Custom LLMs can be tailored to address the specific pain points of customers, leading to enhanced user satisfaction. For instance, a fine-tuned model can provide more relevant answers to queries or assist in troubleshooting specific issues within a particular context, thereby improving overall user engagement.
Applications of Fine-Tuned LLMs
1. Customer Support Automation
Fine-tuned LLMs can revolutionize customer support by automating responses to common queries and issues. By understanding the specific needs of customers in a given industry, these models can provide precise, context-aware responses, significantly reducing response times and improving customer satisfaction.
2. Content Creation and Marketing
Businesses can leverage fine-tuned LLMs for generating high-quality marketing content, blog posts, and social media updates. By training models on brand-specific language and themes, organizations can ensure that their content aligns with their voice and resonates with their audience.
3. Data Analysis and Insights
Custom LLMs can analyze large volumes of unstructured data, extracting valuable insights that drive business decisions. By fine-tuning models to understand industry-specific data patterns and trends, organizations can make more informed, data-driven choices.
Steps to Build and Fine-Tune LLMs
1. Define Clear Objectives
Before starting the fine-tuning process, it’s essential to identify the specific goals you want to achieve. Consider what tasks you want the model to perform and the expected outcomes.
2. Gather Relevant Data
Collect datasets that represent the language, terminology, and context relevant to your business. This data will be crucial for training the model effectively.
3. Choose the Right Model
Select a pre-trained language model that best fits your needs. Popular choices include GPT, BERT, and T5., each offering different strengths based on the application.
4. Fine-Tuning the Model
Utilize transfer learning techniques to adapt the chosen model using your collected datasets. This process adjusts the model’s weights to improve its performance on your specific tasks.
5. Testing and Validation
Once the model is fine-tuned, rigorously test it to evaluate its performance. Validate its outputs against expected results, and make adjustments as necessary to ensure reliability and accuracy.
6. Deployment and Monitoring
After successful testing, deploy the fine-tuned model into your production environment. Continually monitor its performance and user feedback to identify areas for further improvement.
Challenges in Fine-Tuning LLMs
1. Data Quality and Availability
The effectiveness of fine-tuning largely depends on the quality and relevance of the training data. Poor quality data can lead to suboptimal model performance, necessitating careful data curation and management.
2. Computational Resources
Training and fine-tuning large models require significant computational power and resources. Organizations must assess their infrastructure capabilities and consider cloud-based solutions if needed.
3. Model Bias and Ethics
Addressing potential biases in language models is critical. Fine-tuned models can inadvertently perpetuate biases present in the training data, necessitating ongoing vigilance and adjustments to promote fairness and inclusivity.
Best Practices for Implementing Custom LLMs
1. Continuous Learning
Keep your models updated with new data and feedback to ensure they remain relevant and effective over time. Continuous learning will enhance their ability to adapt to changing business needs and user expectations.
2. Collaboration with Experts
Engage with NLP and machine learning experts to navigate the complexities of building and fine-tuning LLMs. Their expertise can guide the process and help avoid common pitfalls.
3. Monitor User Feedback
Actively seek user feedback to identify areas for improvement. Regularly assess how well the model meets user needs and make necessary adjustments based on real-world performance.
4. Ethical Considerations
Ensure that the deployment of fine-tuned models aligns with ethical guidelines and best practices. Consider the potential implications of your models on users and society as a whole.
Conclusion
Custom Fine-Tuned LLMs represent a powerful tool for businesses aiming to enhance their operational efficiency and user engagement. By investing in the development of tailored language models, organizations can unlock new possibilities in automation and language understanding, driving innovation and growth.
As technology continues to advance, the potential for fine-tuned LLMs to transform business processes will only increase. Embracing this opportunity will empower companies to stay competitive in a rapidly changing landscape and deliver exceptional value to their customers.